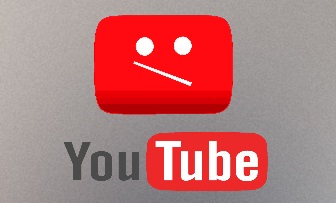
Youtube video for this section is still under creation. Please be patient ^^
Now that you have an Ollama server running and Yacana installed let's create our first agent!
Create a Python file with this content:
from yacana import Agent
agent1 = Agent("AI assistant", "llama3.1:8b", system_prompt="You are a helpful AI assistant", endpoint="http://127.0.0.1:11434")
The Agent()
class takes...
ollama list
to list the
models you have downloaded.
This framework is not meant for basic roleplay. However, for people starting their journey in the realm of AI and for debugging purposes, we added a simple chat system. Add this new line to test it :
agent1.simple_chat()
When running this python file you should enter a chat with the LLM. The Agent keeps track of the history so that it can answer using past information.
$ python3 simple_chat_demo.py
Type 'quit' then enter to exit.
> hey
Hey! It's nice to meet you. Is there something I can help you with, or would you like to chat about something in particular? I'm here to assist you with any questions or topics you'd like to discuss.
>
Let's change the system prompt and have some fun!
agent1 = Agent("Pirate", "llama3.1:8b", system_prompt="You are a pirate", endpoint="http://127.0.0.1:11434")
Type 'quit' then enter to exit.
> hey
Arrrr, shiver me timbers! What be bringin' ye to these fair waters? Are ye lookin' fer a swashbucklin' adventure or just passin' through?
> Searching for the tresor of red beard any idea where it's hidden ?
Red Beard's treasure, ye say? (puffs on pipe) Well, I be knowin' a thing or two about that scurvy dog and his loot. But, I'll only be tellin' ye if ye be willin' to share yer own booty... of information! (winks)
Complete section code:
from yacana import Agent
agent1 = Agent("Pirate", "llama3.1:8b", system_prompt="You are a pirate", endpoint="http://127.0.0.1:11434")
agent1.simple_chat()
⚠️From now on, for clarity, we will not set the endpoint attribute anymore for clarity and will resort to the defaults. If your LLM is not served by Ollama or isn't on your localhost you should continue setting this value.
The whole concept of the framework lies here. If you understand this following section then you have mastered 80% of Yacana's building principle. Like in LangGraph, where you create nodes that you link together, Yacana has a Task() class which takes as arguments a task to solve. There are no hardcoded links between the Tasks so it's easy to refactor and move things around. The important concept to grasp here is that through these Tasks you will give instructions to the LLM in a way that the result must be computable. Meaning instructions must be clear and the prompt to use must reflect that. It's a Task, it's a job, it's something that needs solving but written like it is given as an order! Let's see some examples :
from yacana import Agent, Task
# First, let's make a basic AI agent
agent1 = Agent("AI assistant", "llama3.1:8b", system_prompt="You are a helpful AI assistant")
# Now we create a task and assign the agent1 to the task
task1 = Task(f"Solve the equation 2 + 2 and output the result", agent1)
# For something to happen, you must call the .solve() method on your task.
task1.solve()
What's happening above?
llama3.1:8b
model. You might need to
update that depending on what LLM you downloaded from Ollama ;
For easing the learning curve the default logging level is INFO. It will show what is going on in Yacana. Note that NOT ALL prompts are shown.
The output should look like this:
INFO: [PROMPT]: Solve the equation 2 + 2 and output the result
INFO: [AI_RESPONSE]: The answer to the equation 2 + 2 is... (drumroll please)... 4!
So, the result of solving the equation 2 + 2 is indeed 4.
If your terminal is working normally you should see the task's prompts in green and starting with the '[PROMPT]' string. The LLM's answer should appear purple and start with the [AI_RESPONSE] string.
The Task class takes 2 mandatory parameters:
Many other parameters can be given to a Task. We will see some of them in the following sections of this tutorial. But you can already check out the Task class documentation.
In the above code snippet, we assigned the agent to the Task. So it's the Task that leads the direction that the AI takes. In most other frameworks it's the opposite. You assign work to an existing agent. This reversed way allows to have fine-grained control on each resolution step as the LLM only follows breadcrumbs (the tasks). The pattern will become even more obvious as we get to the Tool section of this tutorial. As you'll see the Tools are also assigned at the Task level and not to the Agent directly.
Compared with LangGraph, we indeed cannot generate a call graph as an image because we don't bind the tasks together explicitly. However, Yacana's way gives more flexibility and allows a hierarchical programming way of scheduling the tasks and keeping control of the flow. It also allows creating new Task dynamically if the need arises. You shall rely on your programming skills and good OOP to have a clean code and good Task ordering. There aren't and will never be any pre-hardcoded interactions and no flat config. This is a framework for developers.
Even though we get logs on the standard output of the terminal, we still need to extract the
answer of the LLM that solved that Task in order to do something with it.
Getting the string message out of it is quite easy as the .solve() method returns a Message() class.
Maybe you are thinking "Ho nooo, another class to deal with". Well, let me tell you
that it's always better to have an OOP class than some semi-random Python dictionary where
you'll forget what keys it contains in a matter of minutes. Also, the Message class is very
straightforward. It exposes a content
attribute. Update the current code to look
like this:
from yacana import Agent, Task, Message
# First, let's make a basic AI agent
agent1 = Agent("AI assistant", "llama3.1:8b", system_prompt="You are a helpful AI assistant")
# Now we create a task and assign the agent1 to the task
task1 = Task(f"Solve the equation 2 + 2 and output the result", agent1)
# So that something actually happens you must call the .solve() method on your task
my_message: Message = task1.solve()
# Printing the LLM's response
print(f"The AI response to our task is : {my_message.content}")
There you go! Give it a try.
Note that we used duck typing to postfix all variables
declaration with their type my_message: Message
. Yacana's source code is entirely
duck-typed so that your IDE always knows what type it's dealing with and proposes the best
methods and arguments. We recommend that you do the same as it's the industry's best standards.
Don't like having 100 lines of code for something simple? Then chain them all in one line!
from yacana import Agent, Task
# First, let's make a basic AI agent
agent1 = Agent("AI assistant", "llama3.1:8b", system_prompt="You are a helpful AI assistant")
# Creating the task, solving it, extracting the result and printing it all in one line
print(f"The AI response to our task is: {Task(f'Solve the equation 2 + 2 and output the result', agent1).solve().content}")
🤔 However, splitting the output of the LLM and the print in two lines would probably look better. Let's not one-line things too much 😅.
For example:
result :str = Task(f'Solve the equation 2 + 2 and output the result', agent1).solve().content
print(f"The AI response to our task is: {result}")
Chaining Tasks is nothing more than just calling a second Task with the same Agent. Agents keep track of the History of what they did (aka, all the Tasks they solved). So just call a second Task and assign the same Agent. For instance, let's multiply by 2 the result of the initial Task. Append this to our current script:
task2_result: str = Task(f'Multiply by 2 our previous result', agent1).solve().content
print(f"The AI response to our second task is : {task2_result}")
You should get:
The AI response to our task is: If we multiply the previous result of 4 by 2, we get:
8
Without tools this only relies on the LLM's ability to do the maths and is dependent on its training.
See? The assigned Agent remembered that it had solved Task1 previously and used this
information to solve the second task.
You can chain as many Tasks as you need. Also, you should create other Agents that don't have
the knowledge of previous tasks and make them do things based on the output of your first agent.
You can build anything now!
As entering the AI landscape can get a bit hairy we decided to leave the INFO log level by
default. This allows to log to the standard output all the requests made to the LLM.
Note that NOT everything of Yacana's internal magic appears in these logs. We don't show
everything because many time-traveling things are going around the history of an Agent and
printing a log at the time it is generated wouldn't always make sense.
However, we try to log a maximum of information to help you understand what is happening
internally and allow you to tweak your prompts accordingly.
Nonetheless, you are the master of what is logged and what isn't. You cannot let Yacana logs
activated when working with an app in production.
There are 5 levels of logs:
"DEBUG"
INFO
DefaultWARNING
ERROR
CRITICAL
None
No logsTo configure the log simply add this line at the start of your program:
from yacana import LoggerManager
LoggerManager.set_log_level("INFO")
Note that Yacana utilizes the Python logging package. This means that setting the level to "DEBUG" will print other libraries' logs on the debug level too.
If you need a library to stop spamming, you can try the following:
from yacana import LoggerManager
LoggerManager.set_library_log_level("httpx", "WARNING")
The above example sets the logging level of the network httpx library to warning, thus reducing the log spamming.
For advanced users, Yacana provides a way to tweak the LLM runtime behavior!
For instance, lowering the temperature
setting makes the model less creative in its
responses. On the contrary, raising this setting will make the LLM more chatty and creative.
Yacana provides you with a class that exposes all the possible LLM properties. Also if you need a
good explanation for each of them I would
recommend the excellent video
Matt Williams did on this subject.
These settings are set at the Agent level so that you can have the same model used by two separate agents and have them behave differently.
We use the ModelSettings class to configure the settings we need.
For example, let's lower the temperature of an Agent to 0.4:
from yacana import ModelSettings, Agent
ms = ModelSettings(temperature=0.4)
agent1 = Agent("Ai assistant", "llama3.1:8b", model_settings=ms)
If you're wondering what the default values of these are when not set. Well, Ollama sets the defaults for you. They can also be overridden in the Model config file (looks like a dockerfile but for LLMs) and finally, you can set them through Yacana during runtime.
A good way to show how this can have a real impact on the output is by setting the
num_predict
parameter. This one allows control of how many tokens should be
generated by the LLM. Let's make the same Task twice but with different num_predict
values:
from yacana import ModelSettings, Agent, Task
# Setting temperature and max token to 100
ms = ModelSettings(temperature=0.4, num_predict=100)
agent1 = Agent("Ai assistant", "llama3.1:8b", model_settings=ms)
Task("Why is the sky blue ?", agent1).solve()
print("-------------------")
# Settings max token to 15
ms = ModelSettings(num_predict=15)
agent2 = Agent("Ai assistant", "llama3.1:8b", model_settings=ms)
Task("Why is the sky blue ?", agent2).solve()
INFO: [PROMPT]: Why is the sky blue ?
INFO: [AI_RESPONSE]: The sky appears blue because of a phenomenon called Rayleigh scattering, named after the British physicist Lord Rayleigh. Here's what happens:
1. **Sunlight**: When sunlight enters Earth's atmosphere, it contains all the colors of the visible spectrum (red, orange, yellow, green, blue, indigo, and violet).
2. **Molecules**: The atmosphere is made up of tiny molecules of gases like nitrogen (N2) and oxygen (O2). These molecules are much smaller than
-------------------
INFO: [PROMPT]: Why is the sky blue ?
INFO: [AI_RESPONSE]: The sky appears blue because of a phenomenon called Rayleigh scattering, named after
As you can see above the two agents didn't output the same number of tokens.